Groupwise: How Google Might Harness Comparisons
BY Dexter Tam
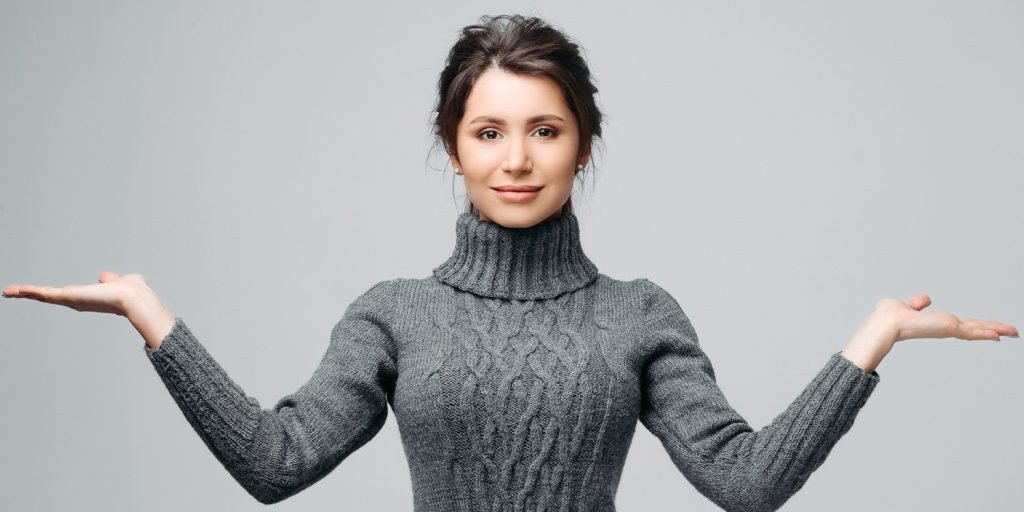
LISTEN
Groupwise scoring functions using deep neural networks: New Google experiment may change the way webpages are ranked.
Deciphering Google’s search algorithms so clients’ sites can perform well on search engine results pages (SERPs) has always been at the heart of a search marketer’s job. However, this is easier said than done. Google never formally announces its ranking factors; it only outlines factors that contribute to a website’s success.
Components that affect page rankings include content, links and on-site search engine optimization (SEO) techniques, such as header tags and meta descriptions.
In the past, Google granted transparency to the masses. PageRank (PR), the first algorithm Google used to rank webpages for its SERPs, was public; you were able to see yours and your competitors’ PRs.
With PageRank, your website was given a score between 1 to 10, with 10 being the best, based on Google’s ranking factors. This allowed search marketers to see tangible changes when they make adjustments to a website. Such transparency, while valued, led to a wide range of manipulations that involved black-hat SEO, or optimization techniques deemed by Google to be unethical, in order to get the best PR score. As a result, Google decided to make PageRank private.
Now, SEO marketers can only make educated guesses about what drives Google’s algorithm and use best practices to ensure their clients’ websites are performing as well as they can by Google’s standards.
How Groupwise might affect rankings
A recently published Google research paper titled: Groupwise Scoring Functions Using Deep Neural Networks may shift everything we know about search ranking and give us better insight about how Google scores websites on its SERPs. Historically, and possibly currently, Google’s machine-learning algorithm graded each webpage in a vacuum, using the ranking factors. It then evaluated the webpage in comparison with its competitors to determine which page is most relevant to the search query. This is known as pointwise scoring function (PSF).
The paper argues that there are limitations to PSF. The example given is a user searching for “Calvin Harris”; most of the search results are tour date information and the latest news. Another user is searching for “Frank Sinatra”; most of the search results are biographies and discographies.
Since Calvin Harris is a relatively new artist, current information is prevalent in SERPs. So if you have an in-depth webpage about Calvin Harris’ life before his fame, chances are you will not show up on the first page. The second example used in the paper, based on previous research, shows that users generally compare the results of the search page before clicking, not just choosing the top result always. This gives credence to the scoring function the authors suggests below, the basis for the research paper.
The authors believe there is a more optimal way of assessing webpages, through an evaluation system called groupwise scoring function (GSF). The authors describe how GSF works by stating, “the relevance score of a document is determined jointly by groups of documents in the list.”
“A GSF takes multiple documents as input and scores them together by leveraging a joint set of features of these documents. It outputs a relative relevance score of a document with respect to the other documents in a group, and the final score of each document is computed by aggregating all the relative relevance scores in a voting mechanism.” Deep neural networks (DNN), a subset of artificial intelligence, and in the same family as machine learning, is used as the scoring function. Deep neural networks are capable of rapidly recognizing patterns within large datasets.
Here is an example of how GSF would work to determine SERP positions. Google’s SERP usually allows 10 organic listings per page. GSF takes these 10 listings, and the DNN scores them as one group, using Google’s ranking factors. The score the DNN produces would be used as the benchmark. Then, the DNN would score each listing individually against the group score and establish each listing’s position.
Using Microsoft Learning to Rank Dataset as the input function, the authors determined that GSF attained considerable performance improvements compared to PSF. Why would GSF perform so well in comparison?
In a PSF, there is no outside or competitor influence shaping the result. It is simply your website against the ranking factors. An appropriate sports analogy is golf. A golfer is playing with other people to win first place, similar to how your website is competing for the top positions on the SERP with other websites. However, in a PSF, other websites — or competing golfers in this analogy — cannot help or hurt your score. You are essentially playing against yourself, with the ranking factors being akin to the par scores in a golf course.
In a GSF, you are contending against your competitors, using a score that is based on all websites together as a baseline. This will give better results in terms of relevancy. It also makes the SERP positions more transparent and fair. It is important to disclose that the authors note their intention for the research paper was to show that GSF could improve relevance in search results, not diversity.
This research paper is significant because if it influences Googles algorithm, search marketers might finally have a guideline into how Google’s algorithm ranks pages in its SERPs. Although Google never publicly goes into detail about what their updates entail, having a Google-sponsored research paper state that their results led to increased performance could mean it has already implemented GSF into its search algorithms. A few search marketing journalists share this sentiment; they believe Google would be prudent to consider implementation, if their own researchers claim GSF can enhance performance.
If GSF is indeed embedded into Google’s search algorithm, the impact it can have in affecting the SERPs for lawyers is significant. Competitor research will be even more important moving forward.
If GSF is indeed used in determining SERP, what can attorneys and their search marketers do to adapt? Go through your target keywords, and see who is ranking on the first page for those terms. What do these sites have in common? How do your competitor’s websites differ from yours? If you find that your website is performing poorly for certain keywords, and can determine a correlation between your competitors’ websites, modifying certain functionalities to mirror your competitors may be beneficial.
LATEST STORIES